82% of enterprises have experimented with AI, but only a fraction successfully scale it. Why?
Many enterprises launch AI pilot projects to streamline operations, enhance customer experience, and boost productivity. But what happens after the pilot? How do you transition AI from an experiment to a full-scale, enterprise-wide solution that delivers measurable, long-term value?
Scaling AI is not just about technology—it’s about strategy, execution, and change management. Whether you’re leveraging AI agents or integrating AI-driven automation, a well-defined approach ensures success. Here’s how you can navigate the AI adoption journey with confidence.
Phased Adoption Strategy: The Roadmap to Success
Jumping straight from a pilot to full-scale production without proper preparation can be risky. A phased approach ensures you can test, iterate, and scale gradually while minimizing disruptions.
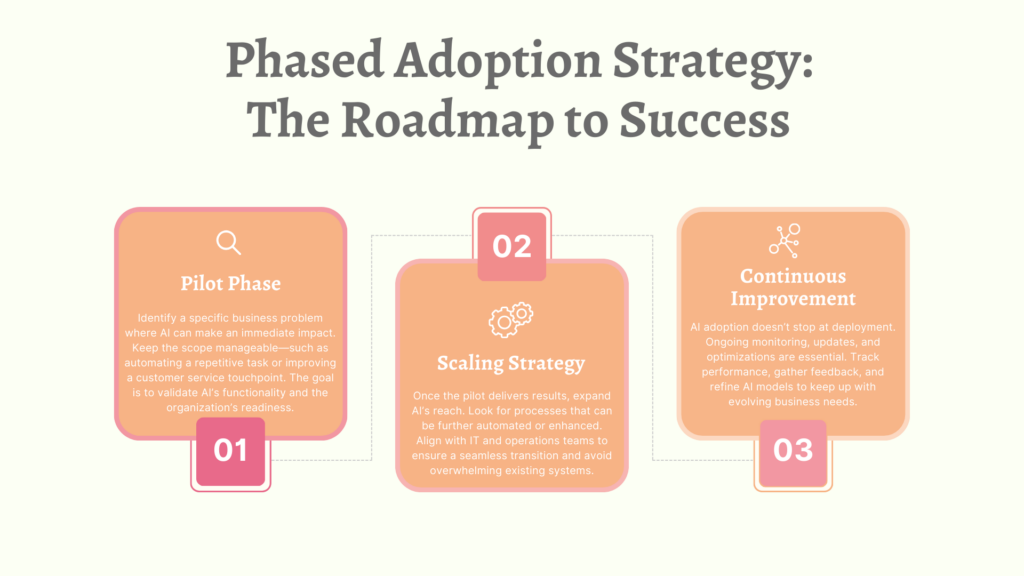
- Pilot Phase: Identify a specific business problem where AI can make an immediate impact. Keep the scope manageable—such as automating a repetitive task or improving a customer service touchpoint. The goal is to validate AI’s functionality and the organization’s readiness.
- Scaling Strategy: Once the pilot delivers results, expand AI’s reach. Look for processes that can be further automated or enhanced. Align with IT and operations teams to ensure a seamless transition and avoid overwhelming existing systems.
- Continuous Improvement: AI adoption doesn’t stop at deployment. Ongoing monitoring, updates, and optimizations are essential. Track performance, gather feedback, and refine AI models to keep up with evolving business needs.
Key Takeaway: Treat AI adoption as an iterative journey, not a one-time deployment. A phased approach ensures you can manage risks while maximizing value at every step.
Overcoming Implementation Challenges: Tackling the Tough Stuff
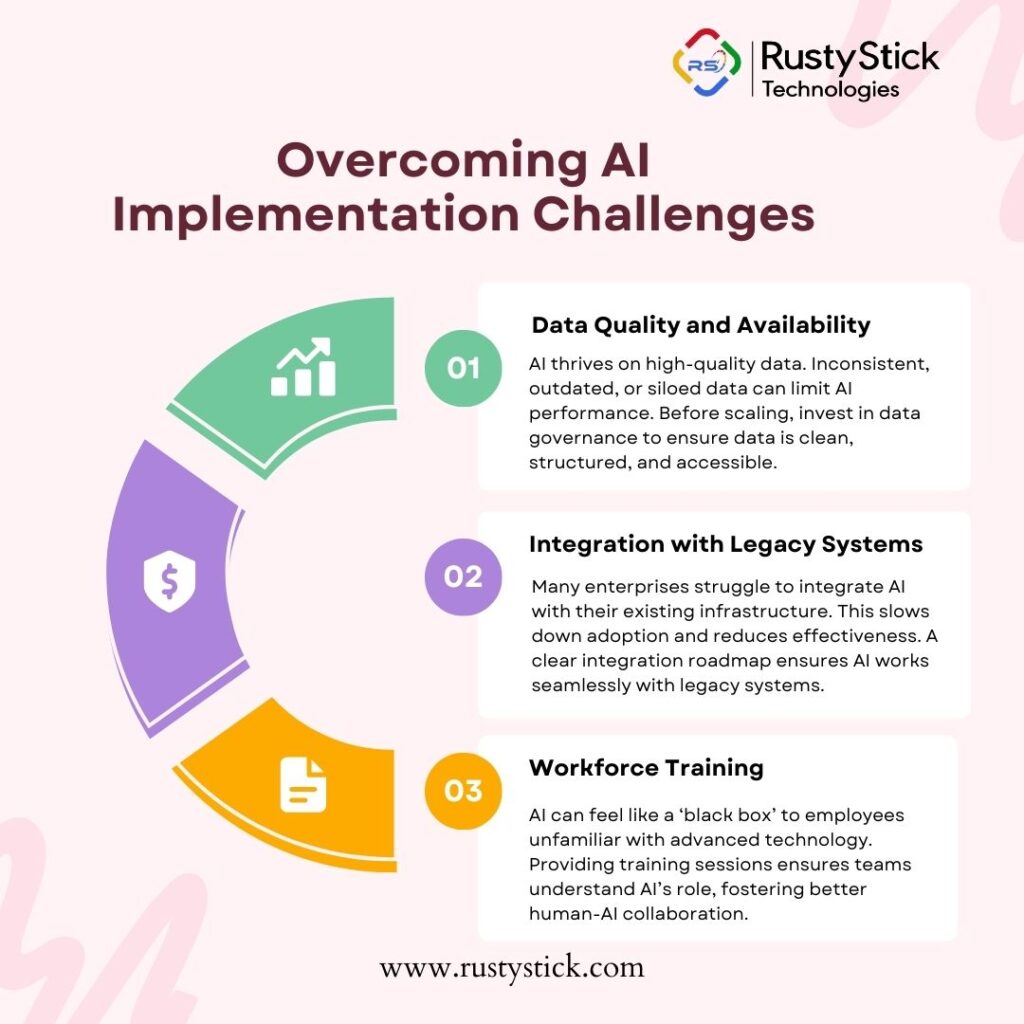
AI adoption comes with its fair share of challenges. The most common hurdles businesses face include:
- Data Quality and Availability: AI thrives on high-quality data. Inconsistent, outdated, or siloed data can limit AI performance. Before scaling, invest in data governance to ensure data is clean, structured, and accessible.
- Integration with Legacy Systems: Many enterprises struggle to integrate AI with their existing infrastructure. This slows down adoption and reduces effectiveness. A clear integration roadmap ensures AI works seamlessly with legacy systems.
- Industry-Specific Challenges: Different industries face unique AI adoption barriers:
- Financial Services: Struggles with regulatory compliance and data security risks.
- Retail: Demand forecasting and personalized customer experiences need real-time AI insights.
- Healthcare: AI adoption is slowed by strict patient data regulations and interoperability issues.
- Workforce Training: AI can feel like a ‘black box’ to employees unfamiliar with advanced technology. Providing training sessions ensures teams understand AI’s role, fostering better human-AI collaboration.
Tip: Overcome these challenges by working with trusted partners who specialize in AI integration, data governance, and workforce training. This is where we, as experts in OutSystems AI solutions, can provide immense value.
Change Management: Ensuring Smooth Transitions
AI implementation impacts employees, processes, and operations. A well-planned change management strategy minimizes friction and accelerates adoption.
Best practices for smooth AI adoption:
- Engage Key Stakeholders Early: Involve IT, operations, and business leaders from the start. Their buy-in ensures AI is successfully integrated into workflows.
- Transparent Communication: Explain why AI is being introduced, its benefits, and its impact on roles. Transparency fosters trust and reduces resistance.
- Provide Support and Resources: Offer ongoing training, dedicated AI support teams, and collaboration spaces for employees to share feedback and resolve issues.
Key Insight: Change management isn’t just about technology—it’s about people. Keeping employees informed and engaged ensures smoother AI adoption.
Measurement and Metrics: How to Track Success
For AI adoption to be truly successful, tracking performance is essential. The right KPIs help assess AI’s impact and justify further investment.
Key AI Adoption Metrics:
- Cost Reduction: How much time and money are saved through automation and efficiency improvements?
- Productivity Gains: Are employees spending less time on repetitive tasks and more on strategic work?
- Customer Satisfaction: Has AI improved response times, personalization, or service quality?
- AI Model Accuracy: Are AI predictions and recommendations aligning with real-world outcomes?
By continuously tracking these KPIs, businesses can optimize AI strategies and ensure long-term success.
Case Studies: Real-World Success Stories
Financial Services Firm: A global financial institution used AI to automate claims processing. After a successful pilot, they scaled the solution across multiple regions, reducing processing time from 5 days to 2 hours and cutting costs by 30%.
Retail Giant: A major retailer optimized inventory management using AI-powered demand forecasting. The result? A 30% reduction in excess stock and improved product availability.
Healthcare Provider: A hospital network introduced AI chatbots to handle patient inquiries. Once the pilot succeeded, they expanded AI to manage appointment bookings, leading to a 15% drop in no-show rates and improved patient engagement.
These real-world examples showcase AI’s transformative power when scaled effectively.
Final Thoughts: Make AI Work for You
Scaling AI from pilot to production isn’t just a technical challenge—it’s a business transformation. By taking a phased approach, overcoming implementation challenges, managing change effectively, and tracking the right KPIs, enterprises can unlock AI’s full potential.